图书介绍
Physical biology of the cell Second editionPDF|Epub|txt|kindle电子书版本网盘下载
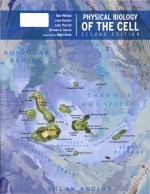
- Rob Phillips; Jane Kondev; Julie Theriot; Hernan G.Garcia; Nigel Orme 著
- 出版社: Garland Science
- ISBN:9780815344506
- 出版时间:2013
- 标注页数:1057页
- 文件大小:238MB
- 文件页数:359页
- 主题词:
PDF下载
下载说明
Physical biology of the cell Second editionPDF格式电子书版下载
下载的文件为RAR压缩包。需要使用解压软件进行解压得到PDF格式图书。建议使用BT下载工具Free Download Manager进行下载,简称FDM(免费,没有广告,支持多平台)。本站资源全部打包为BT种子。所以需要使用专业的BT下载软件进行下载。如BitComet qBittorrent uTorrent等BT下载工具。迅雷目前由于本站不是热门资源。不推荐使用!后期资源热门了。安装了迅雷也可以迅雷进行下载!
(文件页数 要大于 标注页数,上中下等多册电子书除外)
注意:本站所有压缩包均有解压码: 点击下载压缩包解压工具
图书目录
PART 1 THE FACTS OF LIFE1
Chapter 1 Why:Biology by the Numbers3
1.1 BIOLOGICAL CARTOGRAPHY3
1.2 PHYSICAL BIOLOGY OF THE CELL4
Model Building Requires a Substrate of Biological Facts and Physical (or Chemical) Principles5
1.3 THE STUFF OF LIFE5
Organisms Are Constructed from Four Great Classes of Macromolecules6
Nucleic Acids and Proteins Are Polymer Languages with Different Alphabets7
1.4 MODEL BUILDING IN BIOLOGY9
1.4.1 Models as Idealizations9
Biological Stuff Can Be Idealized Using Many Different Physical Models11
1.4.2 Cartoons and Models16
Biological Cartoons Select Those Features of the Problem Thought to Be Essential16
Quantitative Models Can Be Built by Mathematicizing the Cartoons19
1.5 QUANTITATIVE MODELS AND THE POWER OF IDEALIZATION20
1.5.1 On the Springiness of Stuff21
1.5.2 The Toolbox of Fundamental Physical Models22
1.5.3 The Unifying Ideas of Biology23
1.5.4 Mathematical Toolkit25
1.5.5 The Role of Estimates26
1.5.6 On Being Wrong29
1.5.7 Rules of Thumb:Biology by the Numbers30
1.6 SUMMARY AND CONCLUSIONS32
1.7 FURTHER READING32
1.8 REFERENCES33
Chapter 2 What and Where:Construction Plans for Cells and Organisms35
2.1 AN ODE TO E.COLI35
2.1.1 The Bacterial Standard Ruler37
The Bacterium E.coli Will Serve as Our Standard Ruler37
2.1.2 Taking the Molecular Census38
The Cellular Interior Is Highly Crowded,with Mean Spacings Between Molecules That Are Comparable to Molecular Dimensions48
2.1.3 Looking Inside Cells49
2.1.4 Where Does E.coli Fit?51
Biological Structures Exist Over a Huge Range of Scales51
2.2 CELLS AND STRUCTURES WITHIN THEM52
2.2.1 Cells:A Rogue’s Gallery52
Cells Come in a Wide Variety of Shapes and Sizes and with a Huge Range of Functions52
Cells from Humans Have a Huge Diversity of Structure and Function57
2.2.2 The Cellular Interior:Organelles59
2.2.3 Macromolecular Assemblies:The Whole is Greater than the Sum of the Parts63
Macromolecules Come Together to Form Assemblies63
Helical Motifs Are Seen Repeatedly in Molecular Assemblies64
Macromolecular Assemblies Are Arranged in Superstructures65
2.2.4 Viruses as Assemblies66
2.2.5 The Molecular Architecture of Cells:From Protein Data Bank (PDB) Files to Ribbon Diagrams69
Macromolecular Structure Is Characterized Fundamentally by Atomic Coordinates69
Chemical Groups Allow Us to Classify Parts of the Structure of Macromolecules70
2.3 TELESCOPING UP IN SCALE:CELLS DON’T GO IT ALONE72
2.3.1 Multicellularity as One of Evolution’s Great Inventions73
Bacteria Interact to Form Colonies such as Biofilms73
Teaming Up in a Crisis:Lifestyle of Dictyostelium discoideum75
Multicellular Organisms Have Many Distinct Communities of Cells76
2.3.2 Cellular Structures from Tissues to Nerve Networks77
One Class of Multicellular Structures is the Epithelial Sheets77
Tissues Are Collections of Cells and Extracellular Matrix77
Nerve Cells Form Complex,Multicellular Complexes78
2.3.3 Multicellular Organisms78
Cells Differentiate During Development Leading to Entire Organisms78
The Cells of the Nematode Worm,Caenorhabditis Elegans,Have Been Charted,Yielding a Cell-by-Cell Picture of the Organism80
Higher-Level Structures Exist as Colonies of Organisms82
2.4 SUMMARY AND CONCLUSIONS83
2.5 PROBLEMS83
2.6 FURTHER READING84
2.7 REFERENCES85
Chapter 3 When:Stopwatches at Many Scales87
3.1 THE HIERARCHY OF TEMPORAL SCALES87
3.1.1 The Pageant of Biological Processes89
Biological Processes Are Characterized by a Huge Diversity of Time Scales89
3.1.2 The Evolutionary Stopwatch95
3.1.3 The Cell Cycle and the Standard Clock99
The E.coli Cell Cycle Will Serve as Our Standard Stopwatch99
3.1.4 Three Views of Time in Biology105
3.2 PROCEDURAL TIME106
3.2.1 The Machines (or Processes) of the Central Dogma107
The Central Dogma Describes the Processes Whereby the Genetic Information Is Expressed Chemically107
The Processes of the Central Dogma Are Carried Out by Sophisticated Molecular Machines108
3.2.2 Clocks and Oscillators110
Developing Embryos Divide on a Regular Schedule Dictated by an Internal Clock111
Diurnal Clocks Allow Cells and Organisms to Be on Time Everyday111
3.3 RELATIVE TIME114
3.3.1 Checkpoints and the Cell Cycle115
The Eukaryotic Cell Cycle Consists of Four Phases Involving Molecular Synthesis and Organization115
3.3.2 Measuring Relative Time117
Genetic Networks Are Collections of Genes Whose Expression Is Interrelated117
The Formation of the Bacterial Flagellum Is Intricately Organized in Space and Time119
3.3.3 Killing the Cell:The Life Cycles of Viruses120
Viral Life Cycles Include a Series of Self-Assembly Processes121
3.3.4 The Process of Development122
3.4 MANIPULATED TIME125
3.4.1 Chemical Kinetics and Enzyme Turnover125
3.4.2 Beating the Diffusive Speed Limit126
Diffusion Is the Random Motion of Microscopic Particles in Solution127
Diffusion Times Depend upon the Length Scale127
Diffusive Transport at the Synaptic Junction Is the Dynamical Mechanism for Neuronal Communication128
Molecular Motors Move Cargo over Large Distances in a Directed Way129
Membrane-Bound Proteins Transport Molecules from One Side of a Membrane to the Other130
3.4.3 Beating the Replication Limit131
3.4.4 Eggs and Spores:Planning for the Next Generation132
3.5 SUMMARY AND CONCLUSIONS133
3.6 PROBLEMS133
3.7 FURTHER READING136
3.8 REFERENCES136
Chapter 4 Who:“Bless the Little Beasties”137
4.1 CHOOSING A GRAIN OF SAND137
Modern Genetics Began with the Use of Peas as a Model System138
4.1.1 Biochemistry and Genetics138
4.2 HEMOGLOBIN AS A MODEL PROTEIN143
4.2.1 Hemoglobin,Receptor-Ligand Binding,and the Other Bohr143
The Binding of Oxygen to Hemoglobin Has Served as a Model System for Ligand-Receptor Interactions More Generally143
Quantitative Analysis of Hemoglobin Is Based upon Measuring the Fractional Occupancy of the Oxygen-Binding Sites as a Function of Oxygen Pressure144
4.2.2 Hemoglobin and the Origins of Structural Biology144
The Study of the Mass of Hemoglobin Was Central in the Development of Centrifugation145
Structural Biology Has Its Roots in the Determination of the Structure of Hemoglobin145
4.2.3 Hemoglobin and Molecular Models of Disease146
4.2.4 The Rise of Allostery and Cooperativity146
4.3 BACTERIOPHAGES AND MOLECULAR BIOLOGY147
4.3.1 Bacteriophages and the Origins of Molecular Biology148
Bacteriophages Have Sometimes Been Called the “Hydrogen Atoms of Biology”148
Experiments on Phages and Their Bacterial Hosts Demonstrated That Natural Selection Is Operative in Microscopic Organisms148
The Hershey-Chase Experiment Both Confirmed the Nature of Genetic Material and Elucidated One of the Mechanisms of Viral DNA Entry into Cells149
Experiments on Phage T4 Demonstrated the Sequence Hypothesis of Collinearity of DNA and Proteins150
The Triplet Nature of the Genetic Code and DNA Sequencing Were Carried Out on Phage Systems150
Phages Were Instrumental in Elucidating the Existence of mRNA151
General Ideas about Gene Regulation Were Learned from the Study of Viruses as a Model System152
4.3.2 Bacteriophages and Modern Biophysics153
Many Single- Molecule Studies of Molecular Motors Have Been Performed on Motors from Bacteriophages154
4.4 A TALE OF TWO CELLS:E.COLI AS A MODEL SYSTEM154
4.4.1 Bacteria and Molecular Biology154
4.4.2 E.coli and the Central Dogma156
The Hypothesis of Conservative Replication Has Falsifiable Consequences156
Extracts from E.coli Were Used to Perform In Vitro Synthesis of DNA,mRNA,and Proteins157
4.4.3 The lac Operon as the “Hydrogen Atom” of Genetic Circuits157
Gene Regulation in E.coli Serves as a Model for Genetic Circuits in General157
The lac Operon Is a Genetic Network That Controls the Production of the Enzymes Responsible for Digesting the Sugar Lactose158
4.4.4 Signaling and Motility:The Case of Bacterial Chemotaxis159
E.coli Has Served as a Model System for the Analysis of Cell Motility159
4.5 YEAST:FROM BIOCHEMISTRY TO THE CELL CYCLE161
Yeast Has Served as a Model System Leading to Insights in Contexts Ranging from Vitalism to the Functioning of Enzymes to Eukaryotic Gene Regulation161
4.5.1 Yeast and the Rise of Biochemistry162
4.5.2 Dissecting the Cell Cycle162
4.5.3 Deciding Which Way Is Up:Yeast and Polarity164
4.5.4 Dissecting Membrane Traffic166
4.5.5 Genomics and Proteomics167
4.6 FLIES AND MODERN BIOLOGY170
4.6.1 Flies and the Rise of Modern Genetics170
Drosophila melanogaster Has Served as a Model System for Studies Ranging from Genetics to Development to the Functioning of the Brain and Even Behavior170
4.6.2 How the Fly Got His Stripes171
4.7 OF MICE AND MEN173
4.8 THE CASE FOR EXOTICA174
4.8.1 Specialists and Experts174
4.8.2 The Squid Giant Axon and Biological Electricity175
There Is a Steady-State Potential Difference Across the Membrane of Nerve Cells176
Nerve Cells Propagate Electrical Signals and Use Them to Communicate with Each Other176
4.8.3 Exotica Toolkit178
4.9 SUMMARY AND CONCLUSIONS179
4.10 PROBLEMS179
4.11 FURTHER READING181
4.12 REFERENCES183
PART 2 LIFE AT REST185
Chapter 5 Mechanical and Chemical Equilibrium in the Living Cell187
5.1 ENERGY AND THE LIFE OF CELLS187
5.1.1 The Interplay of Deterministic and Thermal Forces189
Thermal Jostling of Particles Must Be Accounted for in Biological Systems189
5.1.2 Constructing the Cell:Managing the Mass and Energy Budget of the Cell190
5.2 BIOLOGICAL SYSTEMS AS MINIMIZERS200
5.2.1 Equilibrium Models for Out of Equilibrium Systems200
Equilibrium Models Can Be Used for Nonequilibrium Problems if Certain Processes Happen Much Faster Than Others201
5.2.2 Proteins in “Equilibrium”202
Protein Structures are Free-Energy Minimizers203
5.2.3 Cells in “Equilibrium”204
5.2.4 Mechanical Equilibrium from a Minimization Perspective204
The Mechanical Equilibrium State is Obtained by Minimizing the Potential Energy204
5.3 THE MATHEMATICS OF SUPERLATIVES209
5.3.1 The Mathematization of Judgement:Functions and Functionals209
Functionals Deliver a Number for Every Function They Are Given210
5.3.2 The Calculus of Superlatives211
Finding the Maximum and Minimum Values of a Function Requires That We Find Where the Slope of the Function Equals Zero211
5.4 CONFIGURATIONAL ENERGY214
In Mechanical Problems,Potential Energy Determines the Equilibrium Structure214
5.4.1 Hooke’s Law:Actin to Lipids216
There is a Linear Relation Between Force and Extension of a Beam216
The Energy to Deform an Elastic Material is a Quadratic Function of the Strain217
5.5 STRUCTURES AS FREE-ENERGY MINIMIZERS219
The Entropy is a Measure of the Microscopic Degeneracy of a Macroscopic State219
5.5.1 Entropy and Hydrophobicity222
Hydrophobicity Results from Depriving Water Molecules of Some of Their Configurational Entropy222
Amino Acids Can Be Classified According to Their Hydrophobicity224
When in Water,Hydrocarbon Tails on Lipids Have an Entropy Cost225
5.5.2 Gibbs and the Calculus of Equilibrium225
Thermal and Chemical Equilibrium are Obtained by Maximizing the Entropy225
5.5.3 Departure from Equilibrium and Fluxes227
5.5.4 Structure as a Competition228
Free Energy Minimization Can Be Thought of as an Alternative Formulation of Entropy Maximization228
5.5.5 An Ode to ΔG230
The Free Energy Reflects a Competition Between Energy and Entropy230
5.6 SUMMARY AND CONCLUSIONS231
5.7 APPENDIX:THE EULER-LAGRANGE EQUATIONS,FINDING THE SUPERLATIVE232
Finding the Extrema of Functionals Is Carried Out Using the Calculus of Variations232
The Euler-Lagrange Equations Let Us Minimize Functionals by Solving Differential Equations232
5.8 PROBLEMS233
5.9 FURTHER READING235
5.10 REFERENCES236
Chapter 6 Entropy Rules!237
6.1 THE ANALYTICAL ENGINE OF STATISTICAL MECHANICS237
The Probability of Different Microstates Is Determined by Their Energy240
6.1.1 A First Look at Ligand-Receptor Binding241
6.1.2 The Statistical Mechanics of Gene Expression:RNA Polymerase and the Promoter244
A Simple Model of Gene Expression Is to Consider the Probability of RNA Polymerase Binding at the Promoter245
Most Cellular RNA Polymerase Molecules Are Bound to DNA245
The Binding Probability of RNA Polymerase to Its Promoter Is a Simple Function of the Number of Polymerase Molecules and the Binding Energy247
6.1.3 Classic Derivation of the Boltzmann Distribution248
The Boltzmann Distribution Gives the Probability of Microstates for a System in Contact with a Thermal Reservoir248
6.1.4 Boltzmann Distribution by Counting250
Different Ways of Partitioning Energy Among Particles Have Different Degeneracies250
6.1.5 Boltzmann Distribution by Guessing253
Maximizing the Entropy Corresponds to Making a Best Guess When Faced with Limited Information253
Entropy Maximization Can Be Used as a Tool for Statistical Inference255
The Boltzmann Distribution is the Maximum Entropy Distribution in Which the Average Energy is Prescribed as a Constraint258
6.2 ON BEING IDEAL259
6.2.1 Average Energy of a Molecule in a Gas259
The Ideal Gas Entropy Reflects the Freedom to Rearrange Molecular Positions and Velocities259
6.2.2 Free Energy of Dilute Solutions262
The Chemical Potential of a Dilute Solution Is a Simple Logarithmic Function of the Concentration262
6.2.3 Osmotic Pressure as an Entropic Spring264
Osmotic Pressure Arises from Entropic Effects264
Viruses,Membrane-Bound Organelles,and Cells Are Subject to Osmotic Pressure265
Osmotic Forces Have Been Used to Measure the Interstrand Interactions of DNA266
6.3 THE CALCULUS OF EQUILIBRIUM APPLIED:LAW OF MASS ACTION267
6.3.1 Law of Mass Action and Equilibrium Constants267
Equilibrium Constants are Determined by Entropy Maximization267
6.4 APPLICATIONS OF THE CALCULUS OF EQUILIBRIUM270
6.4.1 A Second Look at Ligand-Receptor Binding270
6.4.2 Measuring Ligand-Receptor Binding272
6.4.3 Beyond Simple Ligand-Receptor Binding:The Hill Function273
6.4.4 ATP Power274
The Energy Released in ATP Hydrolysis Depends Upon the Concentrations of Reactants and Products275
6.5 SUMMARY AND CONCLUSIONS276
6.6 PROBLEMS276
6.7 FURTHER READING278
6.8 REFERENCES278
Chapter 7 Two-State Systems:From Ion Channels to Cooperative Binding281
7.1 MACROMOLECULES WITH MULTIPLE STATES281
7.1.1 The Internal State Variable Idea281
The State of a Protein or Nucleic Acid Can Be Characterized Mathematically Using a State Variable282
7.1.2 Ion Channels as an Example of Internal State Variables286
The Open Probability (σ) of an Ion Channel Can Be Computed Using Statistical Mechanics287
7.2 STATE VARIABLE DESCRIPTION OF BINDING289
7.2.1 The Gibbs Distribution:Contact with a Particle Reservoir289
The Gibbs Distribution Gives the Probability of Microstates for a System in Contact with a Thermal and Particle Reservoir289
7.2.2 Simple Ligand-Receptor Binding Revisited291
7.2.3 Phosphorylation as an Example of Two Internal State Variables292
Phosphorylation Can Change the Energy Balance Between Active and Inactive States293
Two-Component Systems Exemplify the Use of Phosphorylation in Signal Transduction295
7.2.4 Hemoglobin as a Case Study in Cooperativity298
The Binding Affinity of Oxygen for Hemoglobin Depends upon Whether or Not Other Oxygens Are Already Bound298
A Toy Model of a Dimeric Hemoglobin (Dimoglobin) Illustrate the Idea of Cooperativity298
The Monod-Wyman-Changeux (MWC) Model Provides a Simple Example of Cooperative Binding300
Statistical Models of the Occupancy of Hemoglobin Can Be Written Using Occupation Variables301
There is a Logical Progression of Increasingly Complex Binding Models for Hemoglobin301
7.3 ION CHANNELS REVISITED:LIGAND-GATED CHANNELS AND THE MWC MODEL305
7.4 SUMMARY AND CONCLUSIONS308
7.5 PROBLEMS308
7.6 FURTHER READING310
7.7 REFERENCES310
Chapter 8 Random Walks and the Structure of Macromolecules311
8.1 WHAT IS A STRUCTURE:PDB OR R G?311
8.1.1 Deterministic versus Statistical Descriptions of Structure312
PDB Files Reflect a Deterministic Description of Macromolecular Structure312
Statistical Descriptions of Structure Emphasize Average Size and Shape Rather Than Atomic Coordinates312
8.2 MACROMOLECULES AS RANDOM WALKS312
Random Walk Models of Macromolecules View Them as Rigid Segments Connected by Hinges312
8.2.1 A Mathematical Stupor313
In Random Walk Models of Polymers,EveryMacromolecular Configuration Is Equally Probable313
The Mean Size of a Random Walk Macromolecule Scales as the Square Root of the Number of Segments,?N314
The Probability of a Given Macromolecular State Depends Upon Its Microscopic Degeneracy315
Entropy Determines the Elastic Properties of Polymer Chains316
The Persistence Length Is a Measure of the Length Scale Over Which a Polymer Remains Roughly Straight319
8.2.2 How Big Is a Genome?321
8.2.3 The Geography of Chromosomes322
Genetic Maps and Physical Maps of Chromosomes Describe Different Aspects of Chromosome Structure322
Different Structural Models of Chromatin Are Characterized by the Linear Packing Density of DNA323
Spatial Organization of Chromosomes Shows Elements of Both Randomness and Order324
Chromosomes Are Tethered at Different Locations325
Chromosome Territories Have Been Obsered in Bacterial Cells327
Chromosome Territories in Vibrio cholerae Can Be Explored Using Models of Polymer Confinement and Tethering328
8.2.4 DNA Looping:From Chromosomes to Gene Regulation333
The Lac Repressor Molecule Acts Mechanistically by Forming a Sequestered Loop in DNA334
Looping of Large DNA Fragments Is Dictated by the Difficulty of Distant Ends Finding Each Other334
Chromosome Conformation Capture Reveals the Geometry of Packing of Entire Genomes in Cells336
8.3 THE NEW WORLD OF SINGLE-MOLECULE MECHANICS337
Single-Molecule Measurement Techniques Lead to Force Spectroscopy337
8.3.1 Force-Extension Curves:A New Spectroscopy339
Different Macromolecules Have Different Force Signatures When Subjected to Loading339
8.3.2 Random Walk Models for Force-Extension Curves340
The Low-Force Regime in Force-Extension Curves Can Be Understood Using the Random Walk Model340
8.4 PROTEINS AS RANDOM WALKS344
8.4.1 Compact Random Walks and the Size of Proteins345
The Compact Nature of Proteins Leads to an Estimate of Their Size345
8.4.2 Hydrophobic and Polar Residues:The HP Model346
The HP Model Divides Amino Acids into Two Classes:Hydrophobic and Polar346
8.4.3 HP Models of Protein Folding348
8.5 SUMMARY AND CONCLUSIONS351
8.6 PROBLEMS351
8.7 FURTHER READING353
8.8 REFERENCES353
Chapter 9 Electrostatics for Salty Solutions355
9.1 WATER AS LIFE’S AETHER355
9.2 THE CHEMISTRY OF WATER358
9.2.1 pH and the Equilibrium Constant358
Dissociation of Water Molecules Reflects a Competition Between the Energetics of Binding and the Entropy of Charge Liberation358
9.2.2 The Charge on DNA and Proteins359
The Charge State of Biopolymers Depends upon the pH of the Solution359
Different Amino Acids Have Different Charge States359
9.2.3 Salt and Binding360
9.3 ELECTROSTATICS FOR SALTY SOLUTIONS360
9.3.1 An Electrostatics Primer361
A Charge Distribution Produces an Electric Field Throughout Space362
The Flux of the Electric Field Measures the Density of Electric Field Lines363
The Electrostatic Potential Is an Alternative Basis for Describing the Electrical State of a System364
There Is an Energy Cost Associated With Assembling a Collection of Charges367
The Energy to Liberate Ions from Molecules Can Be Comparable to the Thermal Energy368
9.3.2 The Charged Life of a Protein369
9.3.3 The Notion of Screening:Electrostatics in Salty Solutions370
Ions in Solution Are Spatially Arranged to Shield Charged Molecules Such as DNA370
The Size of the Screening Cloud Is Determined by a Balance of Energy and Entropy of the Surrounding Ions371
9.3.4 The Poisson-Boltzmann Equation374
The Distribution of Screening Ions Can Be Found by Minimizing the Free Energy374
The Screening Charge Decays Exponentially Around Macromolecules in Solution376
9.3.5 Viruses as Charged Spheres377
9.4 SUMMARY AND CONCLUSION379
9.5 PROBLEMS380
9.6 FURTHER READING382
9.7 REFERENCES382
Chapter 10 Beam Theory:Architecture for Cells and Skeletons383
10.1 BEAMS ARE EVERYWHERE:FROM FLAGELLA TO THE CYTOSKELETON383
One-Dimensional Structural Elements Are the Basis of Much of Macromolecular and Cellular Architecture383
10.2 GEOMETRY AND ENERGETICS OF BEAM DEFORMATION385
10.2.1 Stretch,Bend,and Twist385
Beam Deformations Result in Stretching,Bending,and Twisting385
A Bent Beam Can Be Analyzed as a Collection of Stretched Beams385
The Energy Cost to Deform a Beam Is a Quadratic Function of the Strain387
10.2.2 Beam Theory and the Persistence Length:Stiffness is Relative389
Thermal Fluctuations Tend to Randomize the Orientation of Biological Polymers389
The Persistence Length Is the Length Over Which a Polymer Is Roughly Rigid390
The Persistence Length Characterizes the Correlations in the Tangent Vectors at Different Positions Along the Polymer390
The Persistence Length Is Obtained by Averaging Over All Configurations of the Polymer391
10.2.3 Elasticity and Entropy:The Worm-Like Chain392
The Worm-Like Chain Model Accounts for Both the Elastic Energy and Entropy of Polymer Chains392
10.3 THE MECHANICS OF TRANSCRIPTIONAL REGULATION:DNA LOOPING REDUX394
10.3.1 The Iac Operon and Other Looping Systems394
Transcriptional Regulation Can Be Effected by DNA Looping395
10.3.2 Energetics of DNA Looping395
10.3.3 Putting It All Together:The J-Factor396
10.4 DNA PACKING:FROM VIRUSES TO EUKARYOTES398
The Packing of DNA in Viruses and Cells Requires Enormous Volume Compaction398
10.4.1 The Problem of Viral DNA Packing400
Structural Biologists Have Determined the Structure of Many Parts in the Viral Parts List400
The Packing of DNA in Viruses Results in a Free-Energy Penalty402
A Simple Model of DNA Packing in Viruses Uses the Elastic Energy of Circular Hoops403
DNA Self-Interactions Are also Important in Establishing the Free Energy Associated with DNA Packing in Viruses404
DNA Packing in Viruses Is a Competition Between Elastic and Interaction Energies406
10.4.2 Constructing the Nucleosome407
Nucleosome Formation Involves Both Elastic Deformation and Interactions Between Histones and DNA408
10.4.3 Equilibrium Accessibility of Nucleosomal DNA409
The Equilibrium Accessibility of Sites within the Nucleosome Depends upon How Far They Are from the Unwrapped Ends409
10.5 THE CYTOSKELETON AND BEAM THEORY413
Eukaryotic Cells Are Threaded by Networks of Filaments413
10.5.1 The Cellular Interior:A Structural Perspective414
Prokaryotic Cells Have Proteins Analogous to the Eukaryotic Cytoskeleton416
10.5.2 Stiffness of Cytoskeletal Filaments416
The Cytoskeleton Can Be Viewed as a Collection of Elastic Beams416
10.5.3 Cytoskeletal Buckling419
A Beam Subject to a Large Enough Force Will Buckle419
10.5.4 Estimate of the Buckling Force420
Beam Buckling Occurs at Smaller Forces for Longer Beams420
10.6 SUMMARY AND CONCLUSIONS421
10.7 APPENDIX:THE MATHEMATICS OF THE WORM-LIKE CHAIN421
10.8 PROBLEMS424
10.9 FURTHER READING426
10.10 REFERENCES426
Chapter 11 Biological Membranes:Life in Two Dimensions427
11.1 THE NATURE OF BIOLOGICAL MEMBRANES427
11.1.1 Cells and Membranes427
Cells and Their Organelles Are Bound by Complex Membranes427
Electron Microscopy Provides a Window on Cellular Membrane Structures429
11.1.2 The Chemistry and Shape of Lipids431
Membranes Are Built from a Variety of Molecules That Have an Ambivalent Relationship with Water431
The Shapes of Lipid Molecules Can Induce Spontaneous Curvature on Membranes436
11.1.3 The Liveliness of Membranes436
Membrane Proteins Shuttle Mass Across Membranes437
Membrane Proteins Communicate Information Across Membranes439
Specialized Membrane Proteins Generate ATP439
Membrane Proteins Can Be Reconstituted in Vesicles439
11.2 ON THE SPRINGINESS OF MEMBRANES440
11.2.1 An Interlude on Membrane Geometry440
Membrane Stretching Geometry Can Be Described by a Simple Area Function441
Membrane Bending Geometry Can Be Described by a Simple Height Function,h(x,y)441
Membrane Compression Geometry Can Be Described by a Simple Thickness Function,w(x,y)444
Membrane Shearing Can Be Described by an Angle Variable,θ444
11.2.2 Free Energy of Membrane Deformation445
There Is a Free-Energy Penalty Associated with Changing the Area of a Lipid Bilayer445
There Is a Free-Energy Penalty Associated with Bending a Lipid Bilayer446
There Is a Free-Energy Penalty for Changing the Thickness of a Lipid Bilayer446
There Is an Energy Cost Associated with the Gaussian Curvature447
11.3 STRUCTURE,ENERGETICS,AND FUNCTION OF VESICLES448
11.3.1 Measuring Membrane Stiffness448
Membrane Elastic Properties Can Be Measured by Stretching Vesicles448
11.3.2 Membrane Pulling450
11.3.3 Vesicles in Cells453
Vesicles Are Used for a Variety of Cellular Transport Processes453
There Is a Fixed Free-Energy Cost Associated with Spherical Vesicles of All Sizes455
Vesicle Formation Is Assisted by Budding Proteins456
There Is an Energy Cost to Disassemble Coated Vesicles458
11.4 FUSION AND FISSION458
11.4.1 Pinching Vesicles:The Story of Dynamin459
11.5 MEMBRANES AND SHAPE462
11.5.1 The Shapes of Organelles462
The Surface Area of Membranes Due to Pleating Is So Large That Organelles Can Have Far More Area than the Plasma Membrane463
11.5.2 The Shapes of Cells465
The Equilibrium Shapes of Red Blood Cells Can Be Found by Minimizing the Free Energy466
11.6 THE ACTIVE MEMBRANE467
11.6.1 Mechanosensitive Ion Channels and Membrane Elasticity467
Mechanosensitive Ion Channels Respond to Membrane Tension467
11.6.2 Elastic Deformations of Membranes Produced by Proteins468
Proteins Induce Elastic Deformations in the Surrounding Membrane468
Protein-Induced Membrane Bending Has an Associated Free-Energy Cost469
11.6.3 One-Dimensional Solution for MscL470
Membrane Deformations Can Be Obtained by Minimizing the Membrane Free Energy470
The Membrane Surrounding a Channel Protein Produces a Line Tension472
11.7 SUMMARY AND CONCLUSIONS475
11.8 PROBLEMS476
11.9 FURTHER READING479
11.10 REFERENCES479
PART 3 LIFE IN MOTION481
Chapter 12 The Mathematics of Water483
12.1 PUTTING WATER IN ITS PLACE483
12.2 HYDRODYNAMICS OF WATER AND OTHER FLUIDS484
12.2.1 Water as a Continuum484
Though Fluids Are Composed of Molecules It Is Possible to Treat Them as a Continuous Medium484
12.2.2 What Can Newton Tell Us?485
Gradients in Fluid Velocity Lead to Shear Forces485
12.2.3 F= ma for Fluids486
12.2.4 The Newtonian Fluid and the Navier-Stokes Equations490
The Velocity of Fluids at Surfaces Is Zero491
12.3 THE RIVER WITHIN:FLUID DYNAMICS OF BLOOD491
12.3.1 Boats in the River:Leukocyte Rolling and Adhesion493
12.4 THE LOW REYNOLDS NUMBER WORLD495
12.4.1 Stokes Flow:Consider a Spherical Bacterium495
12.4.2 Stokes Drag in Single-Molecule Experiments498
Stokes Drag Is Irrelevant for Optical Tweezers Experiments498
12.4.3 Dissipative Time Scales and the Reynolds Number499
12.4.4 Fish Gotta Swim,Birds Gotta Fly,and Bacteria Gotta Swim Too500
Reciprocal Deformation of the Swimmer’s Body Does Not Lead to Net Motion at Low Reynolds Number502
12.4.5 Centrifugation and Sedimentation:Spin It Down502
12.5 SUMMARY AND CONCLUSIONS504
12.6 PROBLEMS505
12.7 FURTHER READING507
12.8 REFERENCES507
Chapter 13 A Statistical View of Biological Dynamics509
13.1 DIFFUSION IN THE CELL509
13.1.1 Active versus Passive Transport510
13.1.2 Biological Distances Measured in Diffusion Times511
The Time It Takes a Diffusing Molecule to Travel a Distance L Grows as the Square of the Distance512
Diffusion Is Not Effective Over Large Cellular Distances512
13.1.3 Random Walk Redux514
13.2 CONCENTRATION FIELDS AND DIFFUSIVE DYNAMICS515
Fick’s Law Tells Us How Mass Transport Currents Arise as a Result of Concentration Gradients517
The Diffusion Equation Results from Fick’s Law and Conservation of Mass518
13.2.1 Diffusion by Summing Over Microtrajectories518
13.2.2 Solutions and Properties of the Diffusion Equation524
Concentration Profiles Broaden Over Time in a Very Precise Way524
13.2.3 FRAP and FCS525
13.2.4 Drunks on a Hill:The Smoluchowski Equation529
13.2.5 The Einstein Relation530
13.3 DIFFUSION TO CAPTURE532
13.3.1 Modeling the Cell Signaling Problem532
Perfect Receptors Result in a Rate of Uptake 4πDcoa533
A Distribution of Receptors Is Almost as Good as a Perfectly Absorbing Sphere534
Real Receptors Are Not Always Uniformly Distributed536
13.3.2 A “Universal” Rate for Diffusion-Limited Chemical Reactions537
13.4 SUMMARY AND CONCLUSIONS538
13.5 PROBLEMS539
13.6 FURTHER READING540
13.7 REFERENCES540
Chapter 14 Life in Crowded and Disordered Environments543
14.1 CROWDING,LINKAGE,AND ENTANGLEMENT543
14.1.1 The Cell Is Crowded544
14.1.2 Macromolecular Networks:The Cytoskeleton and Beyond545
14.1.3 Crowding on Membranes546
14.1.4 Consequences of Crowding547
Crowding Alters Biochemical Equilibria548
Crowding Alters the Kinetics within Cells548
14.2 EQUILIBRIA IN CROWDED ENVIRONMENTS550
14.2.1 Crowding and Binding550
Lattice Models of Solution Provide a Simple Picture of the Role of Crowding in Biochemical Equilibria550
14.2.2 Osmotic Pressures in Crowded Solutions552
Osmotic Pressure Reveals Crowding Effects552
14.2.3 Depletion Forces:Order from Disorder554
The Close Approach of Large Particles Excludes Smaller Particles Between Them,Resulting in an Entropic Force554
Depletion Forces Can Induce Entropic Ordering!559
14.2.4 Excluded Volume and Polymers559
Excluded Volume Leads to an Effective Repulsion Between Molecules559
Self-avoidance Between the Monomers of a Polymer Leads to Polymer Swelling561
14.2.5 Case Study in Crowding:How to Make a Helix563
14.2.6 Crowding at Membranes565
14.3 CROWDED DYNAMICS566
14.3.1 Crowding and Reaction Rates566
Enzymatic Reactions in Cells Can Proceed Faster than the Diffusion Limit Using Substrate Channeling566
Protein Folding Is Facilitated by Chaperones567
14.3.2 Diffusion in Crowded Environments567
14.4 SUMMARY AND CONCLUSIONS569
14.5 PROBLEMS569
14.6 FURTHER READING570
14.7 REFERENCES571
Chapter 15 Rate Equations and Dynamics in the Cell573
15.1 BIOLOGICAL STATISTICAL DYNAMICS:A FIRST LOOK573
15.1.1 Cells as Chemical Factories574
15.1.2 Dynamics of the Cytoskeleton575
15.2 A CHEMICAL PICTURE OF BIOLOGICAL DYNAMICS579
15.2.1 The Rate Equation Paradigm579
Chemical Concentrations Vary in Both Space and Time580
Rate Equations Describe the Time Evolution of Concentrations580
15.2.2 All Good Things Must End581
Macromolecular Decay Can Be Described by a Simple,First-Order Differential Equation581
15.2.3 A Single-Molecule View of Degradation:Statistical Mechanics Over Trajectories582
Molecules Fall Apart with a Characteristic Lifetime582
Decay Processes Can Be Described with Two-State Trajectories583
Decay of One Species Corresponds to Growth in the Number of a Second Species585
15.2.4 Bimolecular Reactions586
Chemical Reactions Can Increase the Concentration of a Given Species586
Equilibrium Constants Have a Dynamical Interpretation in Terms of Reaction Rates588
15.2.5 Dynamics of Ion Channels as a Case Study589
Rate Equations for Ion Channels Characterize the Time Evolution of the Open and Closed Probability590
15.2.6 Rapid Equilibrium591
15.2.7 Michaelis-Menten and Enzyme Kinetics596
15.3 THE CYTOSKELETON IS ALWAYS UNDER CONSTRUCTION599
15.3.1 The Eukaryotic Cytoskeleton599
The Cytoskeleton Is a Dynamical Structure That Is Always Under Construction599
15.3.2 The Curious Case of the Bacterial Cytoskeleton600
15.4 SIMPLE MODELS OF CYTOSKELETAL POLYMERIZATION602
The Dynamics of Polymerization Can Involve Many Distinct Physical and Chemical Effects603
15.4.1 The Equilibrium Polymer604
Equilibrium Models of Cytoskeletal Filaments Describe the Distribution of Polymer Lengths for Simple Polymers604
An Equilibrium Polymer Fluctuates in Time606
15.4.2 Rate Equation Description of Cytoskeletal Polymerization609
Polymerization Reactions Can Be Described by Rate Equations609
The Time Evolution of the Probability Distribution Pn(t) Can Be Written Using a Rate Equation610
Rates of Addition and Removal of Monomers Are Often Different on the Two Ends of Cytoskeletal Filaments612
15.4.3 Nucleotide Hydrolysis and Cytoskeletal Polymerization614
ATP Hydrolysis Sculpts the Molecular Interface,Resulting in Distinct Rates at the Ends of Cytoskeletal Filaments614
15.4.4 Dynamic Instability:A Toy Model of the Cap615
A Toy Model of Dynamic Instability Assumes That Catastrophe Occurs When Hydrolyzed Nucleotides Are Present at the Growth Front616
15.5 SUMMARY AND CONCLUSIONS618
15.6 PROBLEMS619
15.7 FURTHER READING621
15.8 REFERENCES621
Chapter 16 Dynamics of Molecular Motors623
16.1 THE DYNAMICS OF MOLECULAR MOTORS:LIFE IN THE NOISY LANE623
16.1.1 Translational Motors:Beating the Diffusive Speed Limit625
The Motion of Eukaryotic Cilia and Flagella Is Driven by Translational Motors628
Muscle Contraction Is Mediated by Myosin Motors630
16.1.2 Rotary Motors634
16.1.3 Polymerization Motors:Pushing by Growing637
16.1.4 Translocation Motors:Pushing by Pulling638
16.2 RECTIFIED BROWNIAN MOTION AND MOLECULAR MOTORS639
16.2.1 The Random Walk Yet Again640
Molecular Motors Can Be Thought of as Random Walkers640
16.2.2 The One-State Model641
The Dynamics of a Molecular Motor Can Be Written Using a Master Equation642
The Driven Diff usion Equation Can Be Transformed into an Ordinary Diffusion Equation644
16.2.3 Motor Stepping from a Free-Energy Perspective647
16.2.4 The Two-State Model651
The Dynamics of a Two-State Motor Is Described by Two Coupled Rate Equations651
Internal States Reveal Themselves in the Form of the Waiting Time Distribution654
16.2.5 More General Motor Models656
16.2.6 Coordination of Motor Protein Activity658
16.2.7 Rotary Motors660
16.3 POLYMERIZATION AND TRANSLOCATION AS MOTOR ACTION663
16.3.1 The Polymerization Ratchet663
The Polymerization Ratchet Is Based on a Polymerization Reaction That Is Maintained Out of Equilibrium666
The Polymerization Ratchet Force -Velocity Can Be Obtained by Solving a Driven Diffusion Equation668
16.3.2 Force Generation by Growth670
Polymerization Forces Can Be Measured Directly670
Polymerization Forces Are Used to Center Cellular Structures672
16.3.3 The Translocation Ratchet673
Protein Binding Can Speed Up Translocation through a Ratcheting Mechanism674
The Translocation Time Can Be Estimated by Solving a Driven Diffusion Equation676
16.4 SUMMARY AND CONCLUSIONS677
16.5 PROBLEMS677
16.6 FURTHER READING679
16.7 REFERENCES679
Chapter 17 Biological Electricity and the Hodgkin-Huxley Model681
17.1 THE ROLE OF ELECTRICITY IN CELLS681
17.2 THE CHARGE STATE OF THE CELL682
17.2.1 The Electrical Status of Cells and Their Membranes682
17.2.2 Electrochemical Equilibrium and the Nernst Equation683
Ion Concentration Differences Across Membranes Lead to Potential Differences683
17.3 MEMBRANE PERMEABILITY:PUMPS AND CHANNELS685
A Nonequilibrium Charge Distribution Is Set Up Between the Cell Interior and the External World685
Signals in Cells Are Often Mediated by the Presence of Electrical Spikes Called Action Potentials686
17.3.1 Ion Channels and Membrane Permeability688
Ion Permeability Across Membranes Is Mediated by Ion Channels688
A Simple Two-State Model Can Describe Many of the Features of Voltage Gating of Ion Channels689
17.3.2 Maintaining a Nonequilibrium Charge State691
Ions Are Pumped Across the Cell Membrane Against an Electrochemical Gradient691
17.4 THE ACTION POTENTIAL693
17.4.1 Membrane Depolarization:The Membrane as a Bistable Switch693
Coordinated Muscle Contraction Depends Upon Membrane Depolarization694
A Patch of Cell Membrane Can Be Modeled as an Electrical Circuit696
The Difference Between the Membrane Potential and the Nernst Potential Leads to an Ionic Current Across the Cell Membrane698
Voltage-Gated Channels Result in a Nonlinear Current-Voltage Relation for the Cell Membrane699
A Patch of Membrane Acts as a Bistable Switch700
The Dynamics of Voltage Relaxation Can Be Modeled Using an RC Circuit702
17.4.2 The Cable Equation703
17.4.3 Depolarization Waves705
Waves of Membrane Depolarization Rely on Sodium Channels Switching into the Open State705
17.4.4 Spikes710
17.4.5 Hodgkin-Huxley and Membrane Transport712
Inactivation of Sodium Channels Leads to Propagating Spikes712
17.5 SUMMARY AND CONCLUSIONS714
17.6 PROBLEMS714
17.7 FURTHER READING715
17.8 REFERENCES715
Chapter 18 Light and Life717
18.1 INTRODUCTION718
18.2 PHOTOSYNTHESIS719
Organisms From All Three of the Great Domains of Life Perform Photosynthesis720
18.2.1 Quantum Mechanics for Biology724
Quantum Mechanical Kinematics Describes States of the System in Terms of Wave Functions725
Quantum Mechanical Observables Are Represented by Operators728
The Time Evolution of Quantum States Can Be Determined Using the Schrodinger Equation729
18.2.2 The Particle-in-a-Box Model730
Solutions for the Box of Finite Depth Do Not Vanish at the Box Edges731
18.2.3 Exciting Electrons With Light733
Absorption Wavelengths Depend Upon Molecular Size and Shape735
18.2.4 Moving Electrons From Hither to Yon737
Excited Electrons Can Suffer Multiple Fates737
Electron Transfer in Photosynthesis Proceeds by Tunneling739
Electron Transfer Between Donor and Acceptor Is Gated by Fluctuations of the Environment745
Resonant Transfer Processes in the Antenna Complex Efficiently Deliver Energy to the Reaction Center747
18.2.5 Bioenergetics of Photosynthesis748
Electrons Are Transferred from Donors to Acceptors Within and Around the Cell Membrane748
Water,Water Everywhere,and Not an Electron to Drink750
Charge Separation across Membranes Results in a Proton-Motive Force751
18.2.6 Making Sugar752
18.2.7 Destroying Sugar757
18.2.8 Photosynthesis in Perspective758
18.3 THE VISION THING759
18.3.1 Bacterial “Vision”760
18.3.2 Microbial Phototaxis and Manipulating Cells with Light763
18.3.3 Animal Vision763
There Is a Simple Relationship between Eye Geometry and Resolution765
The Resolution of Insect Eyes Is Governed by Both the Number of Ommatidia and Diffraction Effects768
The Light-Driven Conformational Change of Retinal Underlies Animal Vision769
Information from Photon Detection Is Amplified by a Signal Transduction Cascade in the Photoreceptor Cell773
The Vertebrate Visual System Is Capable of Detecting Single Photons776
18.3.4 Sex,Death,and Quantum Mechanics781
Let There Be Light:Chemical Reactions Can Be Used to Make Light784
18.4 SUMMARY AND CONCLUSIONS785
18.5 APPENDIX:SIMPLE MODEL OF ELECTRON TUNNELING785
18.6 PROBLEMS793
18.7 FURTHER READING795
18.8 REFERENCES796
PART 4 THE MEANING OF LIFE799
Chapter 19 Organization of Biological Networks801
19.1 CHEMICAL AND INFORMATIONAL ORGANIZATION IN THE CELL801
Many Chemical Reactions in the Cell are Linked in Complex Networks801
Genetic Networks Describe the Linkages Between Different Genes and Their Products802
Developmental Decisions Are Made by Regulating Genes802
Gene Expression Is Measured Quantitatively in Terms of How Much,When,and Where804
19.2 GENETIC NETWORKS:DOING THE RIGHT THING AT THE RIGHT TIME807
Promoter Occupancy Is Dictated by the Presence of Regulatory Proteins Called Transcription Factors808
19.2.1 The Molecular Implementation of Regulation:Promoters,Activators,and Repressors808
Repressor Molecules Are the Proteins That Implement Negative Control808
Activators Are the Proteins That Implement Positive Control809
Genes Can Be Regulated During Processes Other Than Transcription809
19.2.2 The Mathematics of Recruitment and Rejection810
Recruitment of Proteins Reflects Cooperativity Between Different DNA-Binding Proteins810
The Regulation Factor Dictates How the Bare RNA Polymerase Binding Probability Is Altered by Transcription Factors812
Activator Bypass Experiments Show That Activators Work by Recruitment813
Repressor Molecules Reduce the Probability Polymerase Will Bind to the Promoter814
19.2.3 Transcriptional Regulation by the Numbers:Binding Energies and Equilibrium Constants819
Equilibrium Constants Can Be Used To Determine Regulation Factors819
19.2.4 A Simple Statistical Mechanical Model of Positive and Negative Regulation820
19.2.5 The Iac Operon822
The Iac Operon Has Features of Both Negative and Positive Regulation822
The Free Energy of DNA Looping Affects the Repression of the Iac Operon824
Inducers Tune the Level of Regulatory Response829
19.2.6 Other Regulatory Architectures829
The Fold-Change for Different Regulatory Motifs Depends Upon Experimentally Accessible Control Parameters830
Quantitative Analysis of Gene Expression in Eukaryotes Can Also Be Analyzed Using Thermodynamic Models832
19.3 REGULATORY DYNAMICS835
19.3.1 The Dynamics of RNA Polymerase and the Promoter835
The Concentrations of Both RNA and Protein Can Be Described Using Rate Equations835
19.3.2 Dynamics of mRNA Distributions838
Unregulated Promoters Can Be Described By a Poisson Distribution841
19.3.3 Dynamics of Regulated Promoters843
The Two-State Promoter Has a Fano Factor Greater Than One844
Different Regulatory Architectures Have Different Fano Factors849
19.3.4 Dynamics of Protein Translation854
19.3.5 Genetic Switches:Natural and Synthetic861
19.3.6 Genetic Networks That Oscillate870
19.4 CELLULAR FAST RESPONSE:SIGNALING872
19.4.1 Bacterial Chemotaxis873
The MWC Model Can Be Used to Describe Bacterial Chemotaxis878
Precise Adaptation Can Be Described by a Simple Balance Between Methylation and Demethylation881
19.4.2 Biochemistry on a Leash883
Tethering Increases the Local Concentration of a Ligand884
Signaling Networks Help Cells Decide When and Where to Grow Their Actin Filaments for Motility884
Synthetic Signaling Networks Permit a Dissection of Signaling Pathways885
19.5 SUMMARY AND CONCLUSIONS888
19.6 PROBLEMS889
19.7 FURTHER READING891
19.8 REFERENCES892
Chapter 20 Biological Patterns:Order in Space and Time893
20.1 INTRODUCTION:MAKING PATTERNS893
20.1.1 Patterns in Space and Time894
20.1.2 Rules for Pattern-Making895
20.2 MORPHOGEN GRADIENTS896
20.2.1 The French Flag Model896
20.2.2 How the Fly Got His Stripes898
Bicoid Exhibits an Exponential Concentration Gradient Along the Anterior-Posterior Axis of Fly Embryos898
A Reaction-Diffusion Mechanism Can Give Rise to an Exponential Concentration Gradient899
20.2.3 Precision and Scaling905
20.2.4 Morphogen Patterning with Growth in Anabaena912
20.3 REACTION-DIFFUSION AND SPATIAL PATTERNS914
20.3.1 Putting Chemistry and Diffusion Together:Turing Patterns914
20.3.2 How Bacteria Lay Down a Coordinate System920
20.3.3 Phyllotaxis:The Art of Flower Arrangement926
20.4 TURNING TIME INTO SPACE:TEMPORAL OSCILLATIONS IN CELL FATE SPECIFICATION931
20.4.1 Somitogenesis932
20.4.2 Seashells Forming Patterns in Space and Time935
20.5 PATTERN FORMATION AS A CONTACT SPORT939
20.5.1 The Notch-Delta Concept939
20.5.2 Drosophila Eyes944
20.6 SUMMARY AND CONCLUSIONS947
20.7 PROBLEMS948
20.8 FURTHER READING949
20.9 REFERENCES950
Chapter 21 Sequences,Specificity,and Evolution951
21.1 BIOLOGICAL INFORMATION952
21.1.1 Why Sequences?953
21.1.2 Genomes and Sequences by the Numbers957
21.2 SEQUENCE ALIGNMENT AND HOMOLOGY960
Sequence Comparison Can Sometimes Reveal Deep Functional and Evolutionary Relationships Between Genes,Proteins,and Organisms961
21.2.1 The HP Model as a Coarse-Grained Model for Bioinformatics964
21.2.2 Scoring Success966
A Score Can Be Assigned to Different Alignments Between Sequences966
Comparison of Full Amino Acid Sequences Requires a 20-by-20 Scoring Matrix968
Even Random Sequences Have a Nonzero Score970
The Extreme Value Distribution Determines the Probability That a Given Alignment Score Would Be Found by Chance971
False Positives Increase as the Threshold for Acceptable Expect Values (also Called E-Values) Is Made Less Stringent973
Structural and Functional Similarity Do Not Always Guarantee Sequence Similarity976
21.3 THE POWER OF SEQUENCE GAZING976
21.3.1 Binding Probabilities and Sequence977
Position Weight Matrices Provide a Map Between Sequence and Binding Affinity978
Frequencies of Nucleotides at Sites Within a Sequence Can Be Used to Construct Position Weight Matrices979
21.3.2 Using Sequence to Find Binding Sites983
21.3.3 Do Nucleosomes Care About Their Positions on Genomes?988
DNA Sequencing Reveals Patterns of Nucleosome Occupancy on Genomes989
A Simple Model Based Upon Self-Avoidance Leads to a Prediction for Nucleosome Positioning990
21.4 SEQUENCES AND EVOLUTION993
21.4.1 Evolution by the Numbers:Hemoglobin and Rhodopsin as Case Studies in Sequence Alignment994
Sequence Similarity Is Used as a Temporal Yardstick to Determine Evolutionary Distances994
Modern-Day Sequences Can Be Used to Reconstruct the Past996
21.4.2 Evolution and Drug Resistance998
21.4.3 Viruses and Evolution1000
The Study of Sequence Makes It Possible to Trace the Evolutionary History of HIV1001
The Luria-Delbruck Experiment Reveals the Mathematics of Resistance1002
21.4.4 Phylogenetic Trees1008
21.5 THE MOLECULAR BASIS OF FIDELITY1010
21.5.1 Keeping It Specific:Beating Thermodynamic Specificity1011
The Specificity of Biological Recognition Often Far Exceeds the Limit Dictated by Free-Energy Differences1011
High Specificity Costs Energy1015
21.6 SUMMARY AND CONCLUSIONS1016
21.7 PROBLEMS1017
21.8 FURTHER READING1020
21.9 REFERENCES1021
Chapter 22 Whither Physical Biology?1023
22.1 DRAWING THE MAP TO SCALE1023
22.2 NAVIGATING WHEN THE MAP IS WRONG1027
22.3 INCREASING THE MAP RESOLUTION1028
22.4 “DIFFICULTIES ON THEORY”1030
Modeler’ s Fantasy1031
Is It Biologically Interesting?1031
Uses and Abuses of Statistical Mechanics1032
Out-of-Equilibrium and Dynamic1032
Uses and Abuses of Continuum Mechanics1032
Too Many Parameters1033
Missing Facts1033
Too Much Stuff1033
Too Little Stuff1034
The Myth of “THE” Cell1034
Not Enough Thinking1035
22.5 THE RHYME AND REASON OF IT ALL1035
22.6 FURTHER READING1036
22.7 REFERENCES1037
Index1039